Deep learning is all the rage these days in enterprise circles, and it isn’t hard to understand why. Whether it is optimizing ad spend, finding new drugs to cure cancer, or just offering better, more intelligent products to customers, machine learning — and particularly deep learning models — have the potential to massively improve a range of products and applications.
The key word though is ‘potential.’ While we have heard oodles of words sprayed across enterprise conferences the last few years about deep learning, there remain huge roadblocks to making these techniques widely available. Deep learning models are highly networked, with dense graphs of nodes that don’t “fit” well with the traditional ways computers process information. Plus, holding all of the information required for a deep learning model can take petabytes of storage and racks upon racks of processors in order to be usable.
There are lots of approaches underway right now to solve this next-generation compute problem, and Cerebras has to be among the most interesting.
As we talked about in August with the announcement of the company’s “Wafer Scale Engine” — the world’s largest silicon chip according to the company — Cerebras’ theory is that the way forward for deep learning is to essentially just get the entire machine learning model to fit on one massive chip. And so the company aimed to go big — really big.
Today, the company announced the launch of its end-user compute product, the Cerebras CS-1, and also announced its first customer of Argonne National Laboratory.
The CS-1 is a “complete solution” product designed to be added to a data center to handle AI workflows. It includes the Wafer Scale Engine (or WSE, i.e. the actual processing core) plus all the cooling, networking, storage, and other equipment required to operate and integrate the processor into the data center. It’s 26.25 inches tall (15 rack units), and includes 400,000 processing cores, 18 gigabytes of on-chip memory, 9 petabytes per second of on-die memory bandwidth, 12 gigabit ethernet connections to move data in and out of the CS-1 system, and sucks just 20 kilowatts of power.
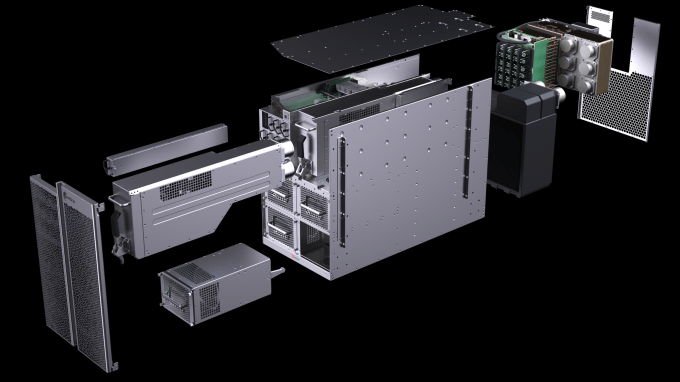
A cross-section look at the CS-1. Photo via Cerebras
Cerebras claims that the CS-1 delivers the performance of more than 1,000 leading GPUs combined — a claim that TechCrunch hasn’t verified, although we are intently waiting for industry-standard benchmarks in the coming months when testers get their hands on these units.
In addition to the hardware itself, Cerebras also announced the release of a comprehensive software platform that allows developers to use popular ML libraries like TensorFlow and PyTorch to integrate their AI workflows with the CS-1 system.
In designing the system, CEO and co-founder Andrew Feldman said that “We’ve talked to more than 100 customers over the past year and a bit,“ in order to determine the needs for a new AI system and the software layer that should go on top of it. “What we’ve learned over the years is that you want to meet the software community where they are rather than asking them to move to you.”
I asked Feldman why the company was rebuilding so much of the hardware to power their system, rather than using already existing components. “If you were to build a Ferrari engine and put it in a Toyota, you cannot make a race car,” Feldman analogized. “Putting fast chips in Dell or [other] servers does not make fast compute. What it does is it moves the bottleneck.” Feldman explained that the CS-1 was meant to take the underlying WSE chip and give it the infrastructure required to allow it to perform to its full capability.
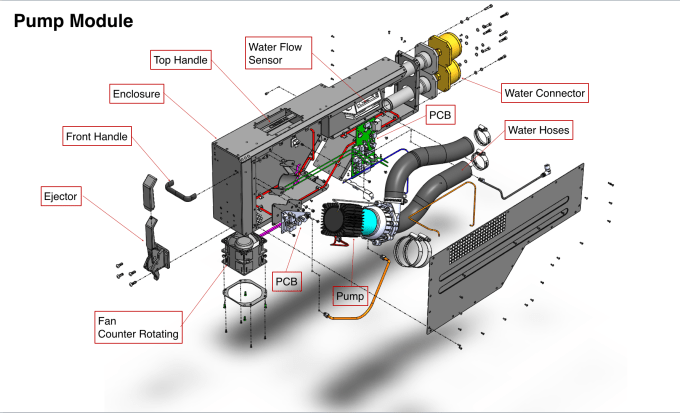
A diagram of the Cerebras CS-1 cooling system. Photo via Cerebras.
That infrastructure includes a high-performance water cooling system to keep this massive chip and platform operating at the right temperatures. I asked Feldman why Cerebras chose water, given that water cooling has traditionally been complicated in the data center. He said, “We looked at other technologies — freon. We looked at immersive solutions, we looked at phase-change solutions. And what we found was that water is extraordinary at moving heat.”
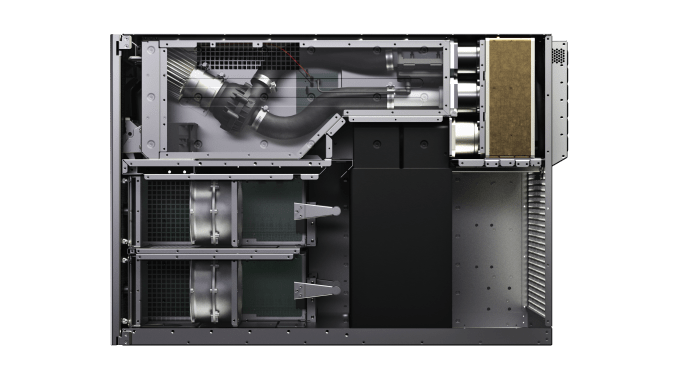
A side view of the CS-1 with its water and air cooling systems visible. Photo via Cerebras.
Why then make such a massive chip, which as we discussed back in August, has huge engineering requirements to operate compared to smaller chips that have better yield from wafers. Feldman said that “ it massively reduces communication time by using locality.”
In computer science, locality is placing data and compute in the right places within, let’s say a cloud, that minimizes delays and processing friction. By having a chip that can theoretically host an entire ML model on it, there’s no need for data to flow through multiple storage clusters or ethernet cables — everything that the chip needs to work with is available almost immediately.
According to a statement from Cerebras and Argonne National Laboratory, Cerebras is helping to power research in “cancer, traumatic brain injury and many other areas important to society today” at the lab. Feldman said that “It was very satisfying that right away customers were using this for things that are important and not for 17-year-old girls to find each other on Instagram or some shit like that.”
(Of course, one hopes that cancer research pays as well as influencer marketing when it comes to the value of deep learning models).
Cerebras itself has grown rapidly, reaching 181 engineers today according to the company. Feldman says that the company is hands down on customer sales and additional product development.
It has certainly been a busy time for startups in the next-generation artificial intelligence workflow space. Graphcore just announced this weekend that it was being installed in Microsoft’s Azure cloud, while I covered the funding of NUVIA, a startup led by the former lead chip designers from Apple who hope to apply their mobile backgrounds to solve the extreme power requirements these AI chips force on data centers.
Expect ever more announcements and activity in this space as deep learning continues to find new adherents in the enterprise.