Photonics is proving to be a tough nut to crack
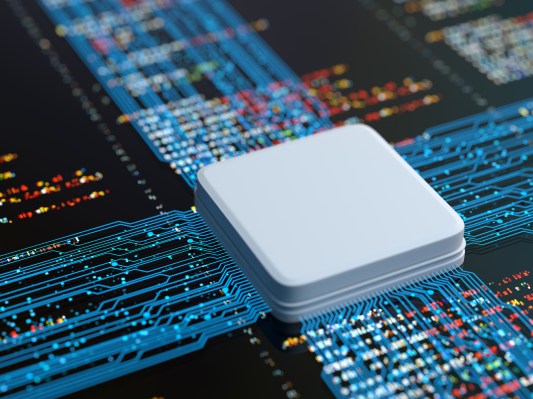
The growing compute power necessary to train sophisticated AI models such as OpenAI’s ChatGPT might eventually run up against a wall with mainstream chip technologies.
In a 2019 analysis, OpenAI found that from 1959 to 2012, the amount of power used to train AI models doubled every two years, and that the power usage began rising seven times faster after 2012.
It’s already causing strain. Microsoft is reportedly facing an internal shortage of the server hardware needed to run its AI, and the scarcity is driving prices up. CNBC, speaking to analysts and technologists, estimates the current cost of training a ChatGPT-like model from scratch to be over $4 million.
One solution to the AI training dilemma that’s been proposed is photonic chips, which use light to send signals rather than the electricity that conventional processors use. Photonic chips could in theory lead to higher training performance because light produces less heat than electricity, can travel faster and is far less susceptible to changes in temperature and electromagnetic fields.
Lightmatter, LightOn, Luminous Computing, Intel and NTT are among the companies developing photonic technologies. But while the technology generated much excitement a few years ago — and attracted a lot of investment — the sector has cooled noticeably since then.
There are various reasons why, but the general message from investors and analysts studying photonics is that photonic chips for AI, while promising, aren’t the panacea they were once believed to be.